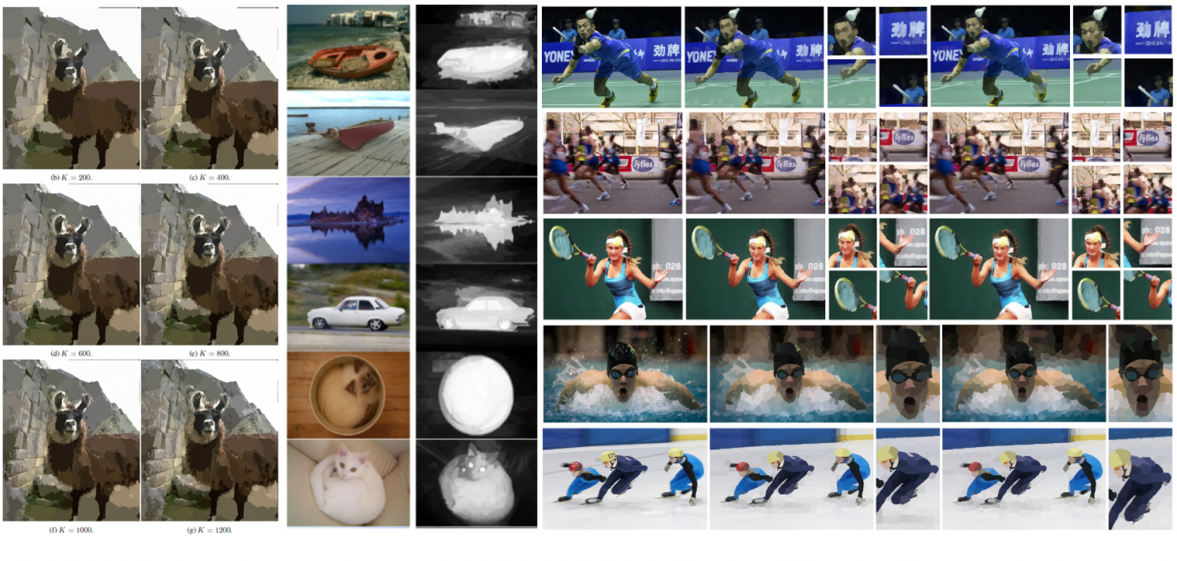
Researcher: Angelica Aviles-Rivero, Hankui Peng, Lihao Liu, and Carola-Bibiane Schönlieb
In recent years unsupervised techniques have become a great focus of attention as they do not require labels. Because labels are expensive, time-consuming and might require expert knowledge. Following this paradigm, clustering algorithms are often used for grouping the image contents with similar characteristics However, these methods are still limited performance-wise as the problems becomes a massively ill-posed problem that needs specific assumptions to mitigate the lack of correspondence between the produced clusters and the known classes. In this project, we explore this family of techniques for image analysis, including in terms of superpixels. Moreover, we also explore techniques different from clustering and propose joint models for improving unsupervised image segmentation, where tasks such as image registration are used to guide the end task. We have explored unsupervised techniques for natural and medical datasets.